How to use artificial intelligence (AI) in your organization
The benefits of AI for organizations are no longer in question. AI supports overall business growth and helps decision-makers to take strategic decisions within short time-frames. In need of talented data engineers and data scientists in order to integrate AI within your company’s operations?
Almost a quarter of the 21stᵉ century has passed, and it is exciting to realize that the key skills increasingly in demand are no longer “just” technical. It is clear that individuals who are able to solve problems are the most valuable. Creative, critical thinkers and good communicators are the future talent of tomorrow.

The undeniable benefits of AI
Artificial Intelligence is no longer a term that requires an introduction. AI gathers a set of techniques and theories that are applied in order to produce machines able to simulate the intelligence of human beings.
The number of companies using AI is constantly growing. For an organization, AI offers multiple benefits:
– Reduction of 25% to 60% in the average time to manage purchasing processes.
– Enables operation 24 hours a day, 7 days a week.
– Elimination and/or reduction of tedious and endless tasks.
– Helps humans with daily-life tasks.
The list is far from exhaustive and extends to all industries and millions of uses and applications.
Companies hunting for AI talent should be aware of an important aspect: AI jobs require mastering of both code and data. This is somewhat different from software developers, who are expected to have “only” code development expertise. Data knowledge is obviously also essential within the job of computer engineer.
AI requires its followers to integrate the following mindset: nothing is certain, everything is uncertain. Which, by the way, can be correlated with the successive crises (sanitary, economic, geopolitical…) that the world has suffered these last 3 years… where the culture of uncertainty seems to overwhelmingly dominate.
The intertwined worlds of AI and Software
Within AI, algorithmic models evolve according to feedback and sensory observations they receive in real-time. This evolution crucially relies on new data that will be integrated in order to determine certain actions. This is precisely how the era of software and the era of AI intersect and cohabit for many years now. However, there are also disparities between AI and software.
Within a software model, we often tend to think that the result of the developed system is certain. The specifications exist, the user stories have been prepared and we know exactly what features the software must contain in order to meet the needs of its end-users.
Within the artificial intelligence model, the result is by nature uncertain. This is ultimately logical, if we deconstruct the term artificial intelligence, which describes a “form of intelligence”, just as human intelligence is also uncertain. Unintentional errors in AI can cause damage and destruction, hence the uncertain nature of the AI paradigm.
The context in the case of the software model is rather “static”. As for the context of AI, it is most definitely “dynamic” in nature.
During software development, we are often using an Agile approach (e.g. the Scrum method). As for coding within AI models, we are often in an experimental framework where we Test & Learn. [Learn more about the concept of Test & Learn in one of our other articles.]
The software paradigm requires engineers or developers who apply the theory, transforming knowledge into practice. In contrast, the AI sector essentially requires scientists who deal with many theoretical concepts.
AI Talents
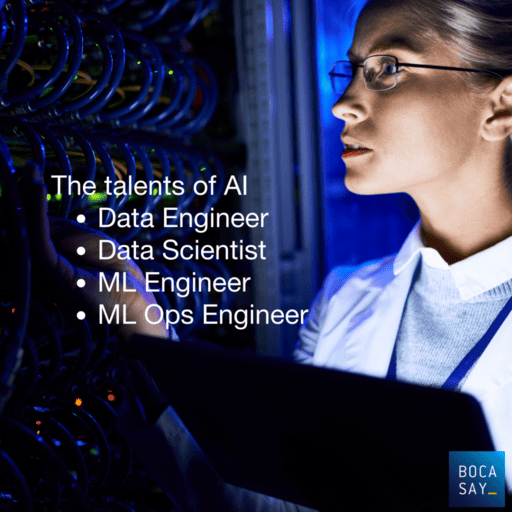
The Data Scientist and the Data Engineer
Data science is an area of expertise with really high demand these days. Indeed, a data scientist has multiple skills that are very useful in a highly technological world: expert in statistics and mathematics and a solid know-how in computer science and big data. A data scientist or engineer is the person that will help a company integrate AI within its operations and objectives. In order to achieve this, he/she interprets the organization’s data while maintaining a global vision.
The data scientist translates business issues into mathematical and statistical issues, able to provide valuable reports that help departments make strategic decisions: including marketing, sales, financial, etc. In other words, he/she has a direct impact on the company as a whole and supports its growth.
The job of a data scientist involves the following tasks:
- Choosing data analysis tools.
- Identifying data storage strategies.
- Collecting and analyzing data that is useful to the business.
- Devising algorithms that will improve search and targeting results.
The Machine-Learning (ML) Engineer
The ML Engineer or Machine Learning Engineer is used to deploy AI at scale. This talent takes over from the Data Scientist. He or she will convert the models into code and deploy them in production so that users can benefit from them. With strong data management skills, ML engineers design software that automates predictive models.
The software designed by the ML Engineer is able to learn by itself, hence the term machine learning. As soon as it performs an operation, it will interpret the results in order to optimize the subsequent operations.
One of the most famous machine learning algorithms is Spotify’s. Every time you listen to a song on this application, the algorithm takes into account your last song selection and will modify its algorithm accordingly. It performs this task in order to be able to offer you song results that are more precise and closer to your previous searches – making each following search better and better.
When it comes to employment, talented experts in AI and Big Data will have nothing to worry about in the future. The brand-new sector of “Big Data Intelligence” increasingly demands a series of job roles:
– Big Data Developer
– Data Miner
– Big data engineer
– Big Data Architect
Reference: The new horizon of digital transformation by Pejman Gohari.